
All healthcare is personal. Individuals have different underlying genetic predispositions, environmental exposures, and past medical histories, not to mention different propensities to engage and respond to treatment and programs.
According to the CDC, precision health not only includes personalized medicine, but also "approaches that occur outside the setting of a doctor's office or hospital, such as disease prevention and health promotion activities." Gone are the days of 'one size fits some' interventions, as the healthcare system moves towards targeted patient care.
The promise of precision health requires assembling a comprehensive, longitudinal view of the patient, potentially incorporating omics, electronic medical records, and social determinants of health data, and accurately predicting disease risk, or adverse outcomes like hospital readmission, with enough time to support an intervention.
Databricks is helping organizations deliver precision health through its Lakehouse Platform, which integrates all types of data with real-time frequency and tooling for the full machine learning (ML) lifecycle. From bespoke care management programs that incorporate web clicks and member engagement data, to behavioral apps that incorporate streaming continuous glucose monitoring data, to personalized medication adherence reminders, to identifying high-risk pregnancies and triggering a workflow for proactive outreach by a nurse, to detecting autism in patients 1.5 years faster, patients are benefiting from care that is tailored to them.
Patient risk scoring
Our latest Solution Accelerator provides the quickstart to predict patient risk and measure quality of care. We start with a robust set of synthetically-generated electronic medical data stored in OMOP 5.3 Common Data Model (Databricks offers another Solution Accelerator around mapping data into the OMOP CDM). The design process includes parameters around the target cohort, the outcome, the observation window, and the risk window.
Given a set of parameters defining the experimental design based on OHDSI best practices for patient-level risk scoring, we create the target and outcome cohorts. For a pre-defined number of comorbidities to consider, comorbidity history, along with demographic features, are added to the feature store. We then use databricks AutoML to train a classifier that predicts the probability of the outcome (in this example, the outcome is emergency room re-admission).
We then register the best model from AutoML in the MLFlow model registry. This model is then used in the next step to predict the risk of admission for a new patient.
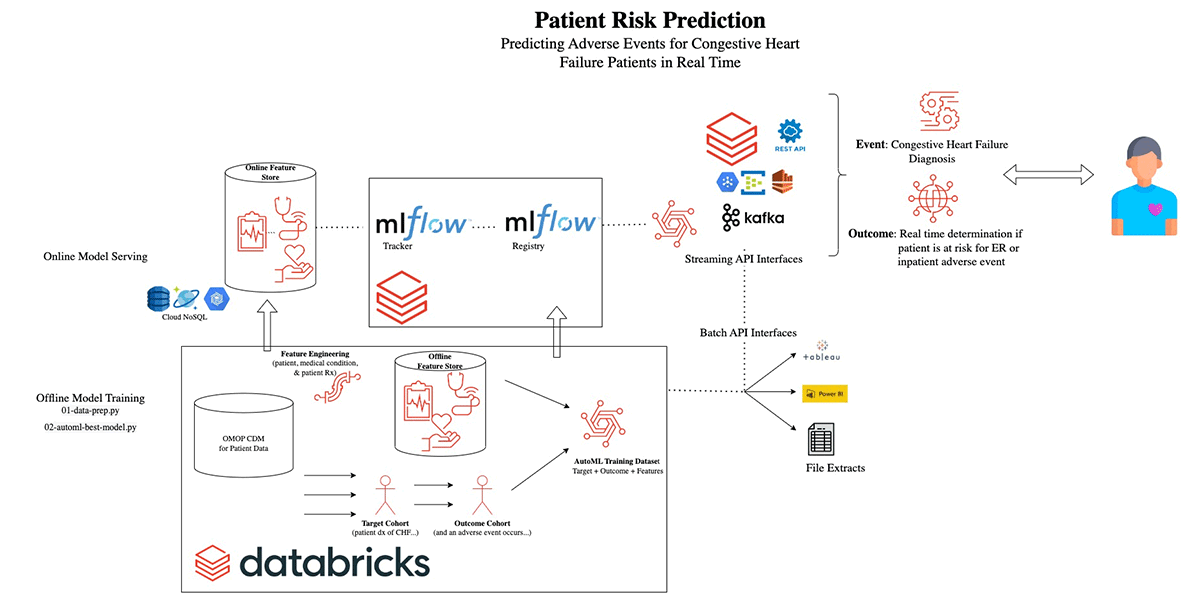
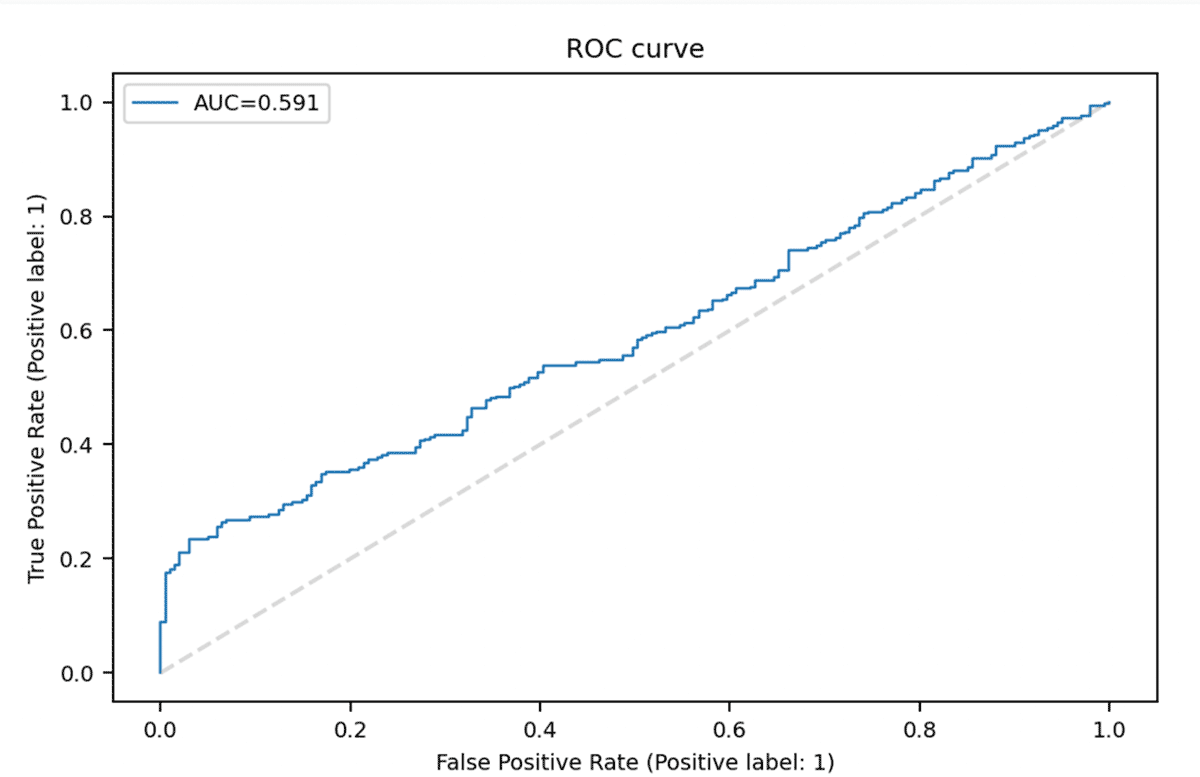
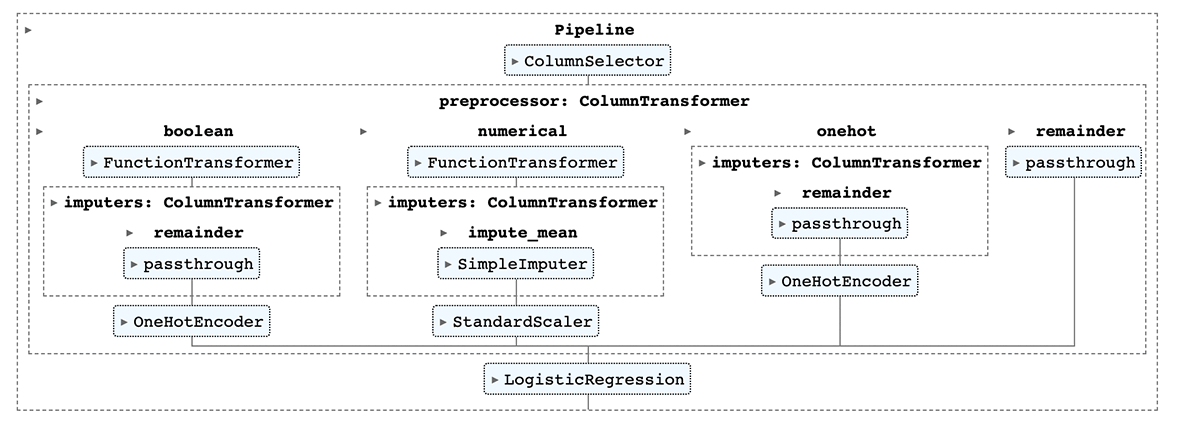
Incorporating quality measures
Building on risk prediction, we next incorporate quality measures. Risk and quality are closely interconnected, and both payers and providers are interested in providing appropriate care in the appropriate settings to reduce waste, increase efficiency, and manage costs. In this example, we look to determine the probability of an Emergency Room (ER) visit for each individual with Congestive Heart Failure (CHF), and identify how quality measures are used to manage this population. The United States Agency for Healthcare Research and Quality (AHRQ) has created a Preventative Indicator Quality Measure as a way to measure an Affordable Care Organization's (ACO) effectiveness at managing patients with CHF.1
AHRQ's CHF measure provides a value between 0 and 1 based on appropriate inpatient vs. outpatient handling of CHF patients (with 0 indicating appropriate management). This measure provides insights for ACOs and payers on unnecessary costs and who is managing care appropriately.
In addition, the AHRQ's CHF measure can be used to encourage members to seek care in high-performing ACOs. Payers can incentivize this behavior with members through Next-Best-Action outreaches to steer members towards high quality care and/or by creating high-performing narrow networks designed for individuals with CHF.